In the last few years, chatbots have dramatically changed the way they operate and provide service to customers. They’ve gone from basic informational interfaces to complex solutions able to communicate with third-party systems.
These changes, together with improvements in technology, have had a significant impact on self-service performance. What does this mean for businesses using an advanced, capable chatbot based on conversational AI?
We, at Inbenta, decided to take a look at our customer’s sessions to detect and analyze self-service trends. The results were quite eye-opening and hope you find the insight useful.
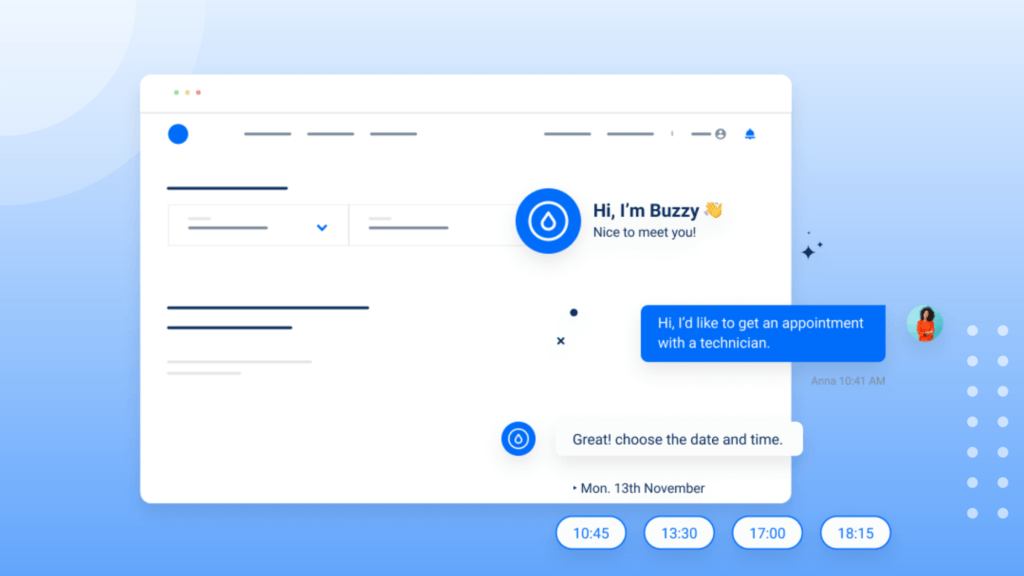
Transactional vs information chatbots. What’s the difference?
Information chatbots are those who are able to identify a request and provide a specific standard answer, whether the answer involves only text, or also images, videos or links to specific pages.
However, although this already allows for a high rate of self-service, and solves a big piece of the customer service puzzle, it still doesn’t cater to more complex, personalized case scenarios.
What happens when users expect an answer that only applies to them personally?
What if they need to achieve or complete a specific action?
Checking their account balance, accessing their medical records, upgrading their insurance policy, rescheduling a flight they’ve booked – a transactional chatbot can handle this kind of more complex requests that require your chatbot to interact with other systems, without having to escalate the conversation to an agent.
These transactions are usually enabled by webhooks and integrations.
We’ve been deploying advanced, transactional chatbots for a while now in highly successful projects. We know transactional projects offer a broader set of options to customers. However, our question was…
How much impact do transactional chatbots have in self-service rates?
We decided to run some research with our data to find out if adding transactionality impacted relevant KPIs in a chatbot project, and if so, by how much.
The first thing we did is take a sample of 4.2 million chatbot conversations from different customers and classify them depending on their nature. They fell into either one of these categories:
- Sessions from chatbots that trigger corporate transactions
- Sessions from chatbots that only provide static information
This would help us draw data and conclusions regarding transactionality.
Once we did that, we took a look at different KPIs, both on a global level and for every category.
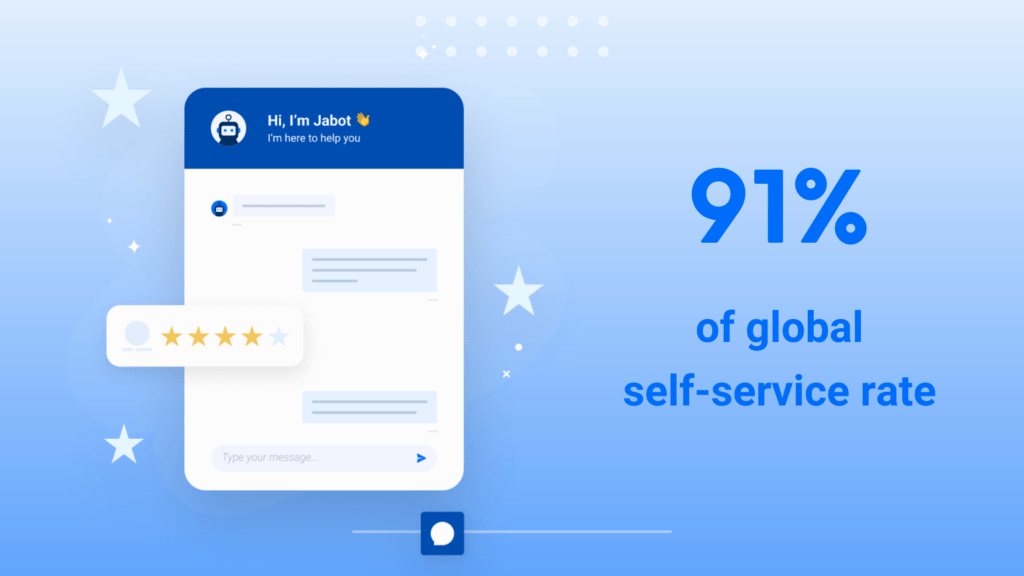
The total self-service rate exceeded 91%
The first thing we noticed was an amazingly high 91% self-service rate, including both transactional and non-transactional instances.
The number doesn’t differ much from our previous tests, which established a typical self-service rate of 90% for our chatbots.
However, it is still pretty impressive, don’t you think? This means that from 4.2 million sessions, only 360K ended up in a contact action. Can you imagine the cost that the remaining 3.8 million customer requests would have had for a customer service department?
Non-transactional chatbots had a lower answer rate
The second thing we noticed was that chatbots with no transactional capabilities had a lower answer rate. This is consistent with the fact that an information chatbot can only cover a certain number of support request cases.
As much as you populate your chatbot with relevant answers to FAQs, if the user seeks to perform an action, and the chatbot isn’t capable of doing so, the result will be an unanswered request.
When analyzing our samples, we saw a 7-point increase in answer rate when using transactional chatbots versus static, information chatbots, which is actually quite outstanding.
Transactional chatbots have a 28% higher self-service rate
The last thing we noticed was that, with transactional chatbots, there were fewer requests ending in contact actions.
We’ve seen that the overall self-service rate of the chatbot was 91%, therefore, only 9% of the total sessions (transactional and non-transactional) reached the support team.
Well, if we compare the sessions from transactional chatbots to those of information chatbots, we can see that the former escalated 28% fewer cases to support.
This proves transactional chatbots offer a better self-service rate and improve overall service. Plus, such a reduction can heavily impact the workload and performance of customer service agents.
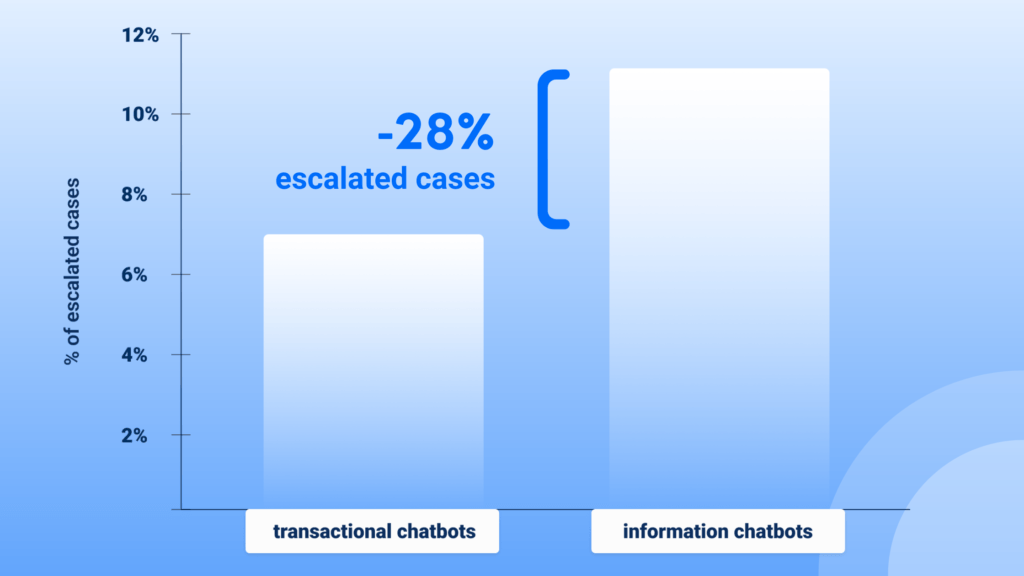
How to analyze the performance of your chatbot instance?
Powerful chatbot data dashboards
Having a robust analytics panel for your chatbot is extremely important in order to follow metrics and KPIs. How else can you know whether your chatbot is performing well or not?
At Inbenta, we’ve built powerful dashboards to help customers understand all the KPIs, for instance:
- Total number of sessions
- Self-service rate
- Unanswered questions
- Escalated sessions
- Other metrics
However, what impact does implementing a transactional chatbot have? In other words, how much adding transactionality reduces operational costs?
ROI on adding transactionality to your chatbot
As you’ve seen, adding transactionality will reduce chatbot escalations by 28%. So if we take it that you had a total of 50,000 chatbot sessions (conversations) a month and 10% of them escalated to a support case, we’re talking of 5,000 support requests. Reducing that by 28% means you’d have 3,600 support requests instead.
Let’s say the average cost per escalated case (phone call, or live assistance) is at 5€.
Adding transactionality to your chatbot would save you 7,000 euros a month.
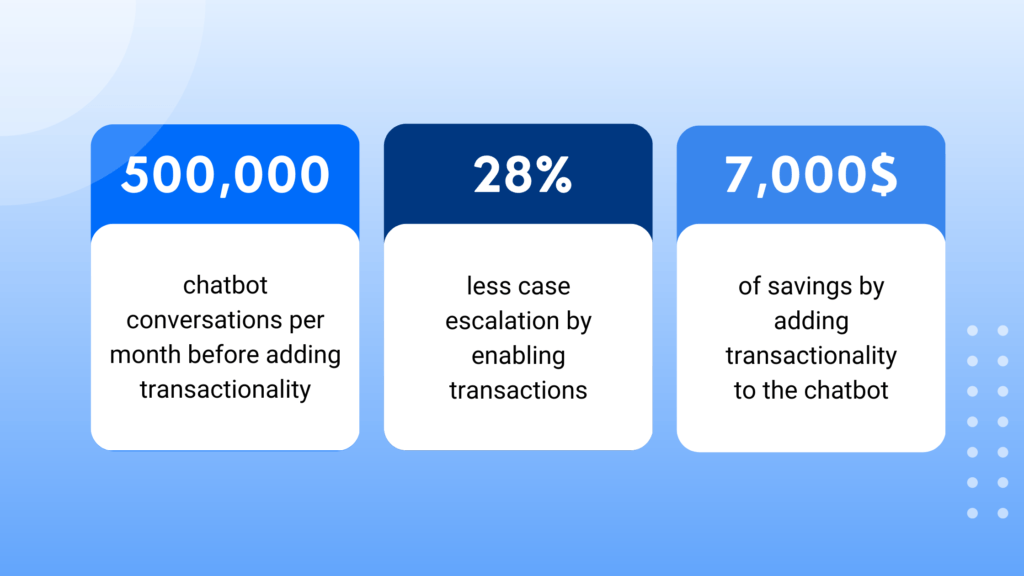
On the other hand, if you run an eCommerce, or any service you can pay for online, enabling transactions can also bring additional revenue to your business.
Let’s say that 5% of the total of chatbot sessions are related to the purchase of a product or a service. Also, let’s estimate the average order value at 50$ (this may vary depending on your business and products).
In that case, the chatbot will handle 2,500 sales a month on its own—can you imagine a shop assistant doing that many sales? It’d mean the sales assistant makes a minimum of 90 sales a day.
These 2,500 monthly sales would account for 125,000 $ of revenue per month. Outstanding, right?
TIP: If your current self-service rate is under 80% to 90%, there is a good chance that your current chatbot or the content it provides is not good enough. If it isn’t transactional, there is also the possibility that your customers are looking to resolve more complex queries an information chatbot can’t tackle.
Making your chatbot transactional
If you’re looking into adding transactionality to your chatbot, you probably need a solution that can easily connect, as well as send and receive data from other platforms.
These can include CRMs, ERPs, HRIS, CMSs and other systems, messaging or social platforms, and other channels, voice-activated or otherwise.
At Inbenta, we’ve been building integrations with well-known platforms for years, and the result is Inbenta’s Apphub. There, you can search and find your favorite apps, connect your Inbenta Chatbot with them and get the most out of your self-service solution.